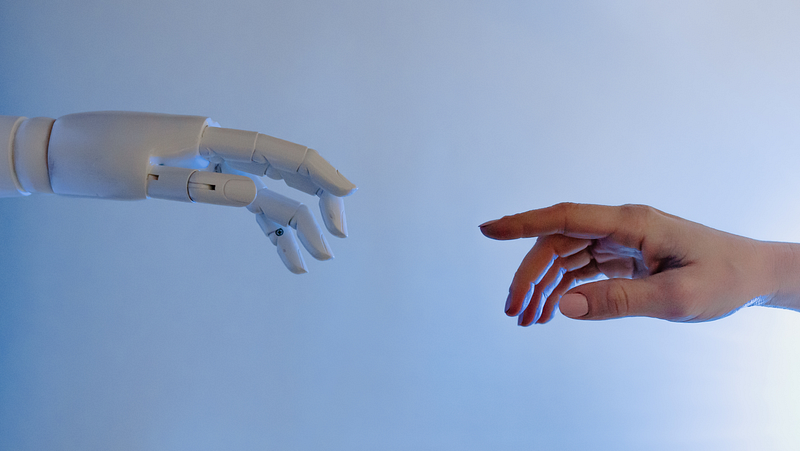
What are Generative Adversarial Networks?
Generative Adversarial Networks (GANs) are a type of artificial intelligence algorithm used to generate new data samples from a training dataset. GANs are made up of two neural networks, a generator and a discriminator, which compete against each other in a game-theoretic setting. The generator network generates new data samples, while the discriminator network evaluates them and tries to determine whether they are real or fake.
The training process for a GAN is an alternating game between the generator and discriminator networks. The generator network first creates a new data sample, which is then passed to the discriminator network. The discriminator network then tries to classify the data sample as real or fake. If the discriminator network correctly classifies the data sample, then the generator network is said to have lost the game. If the discriminator network incorrectly classifies the data sample, then the generator network is said to have won the game.
The goal of the training process is for the generator network to eventually learn how to generate data samples that fool the discriminator network into thinking they are real. Once the generator network has learned to do this, it can be used to generate new data samples that are realistic and useful.
GANs have been used for a variety of tasks, including image generation, video generation, and text generation. They are a powerful tool for artificial intelligence and machine learning, and have shown great promise in many applications.
How do they work?
Generative Adversarial Networks (GANs) are a powerful tool for generating realistic synthetic data. They are made up of two components: a generator network and a discriminator network.
The generator network takes in noise as input and generates data that is realistic enough to fool the discriminator network. The discriminator network takes in both real data and fake data generated by the generator network and tries to classify each instance as either real or fake. This creates a competition between the generator and discriminator networks, where the generator is trying to generate data that is realistic enough to fool the discriminator and the discriminator is trying to become better at identifying fake data. As the training process progresses, the generator network gets better at generating realistic data, and the discriminator network gets better at identifying fake data.
One of the great things about GANs is that they can be used to generate data that is otherwise difficult or impossible to obtain. For example, GANs have been used to generate realistic synthetic images, videos, and even 3D models. This can be incredibly helpful for training machine learning models, as it allows for a much larger dataset to be used for training.
Additionally, GANs can be used to generate data from different distributions than the training data, which can be helpful for exploring the space of possible data distributions.
There are a few things to keep in mind when using GANs
First, GANs can be very sensitive to hyperparameters and during training it can be difficult to achieve convergence between the generator and discriminator.
Second, GANs can be mode collapsed, which means that the generator network only learns to generate a small subset of the possible data. This can be alleviated by using a variety of techniques, such as adding more noise to the input of the generator network or using a different loss function.
Finally, GANs can be difficult to interpret, as there is no obvious way to map the noise input to the generated data.
Overall, GANs are a powerful tool for generating realistic synthetic data. They are relatively new and there is still a lot of active research being done in this area. As more research is done, GANs will become more robust and easier to use, making them an even more powerful tool for data generation.
What are their potential applications in advertising?
In the world of marketing, Generative Adversarial Networks (GANs) are becoming increasingly popular for creating new image ads, text ads, video ads, and audio ads.
GANs have been used to generate realistic images, videos, and even audio recordings. In the realm of marketing, GANs can be used to create new ads that feel more realistic and believable to the end user than those created by traditional methods.
For example, consider a company that sells a product that is designed to reduce wrinkles. The company could use a GAN to create an image ad that shows a person’s face before and after using the product. The before and after images would be generated by the GAN, based on real data samples. The resulting image would be more realistic and believable than an image created by traditional means (e.g. images doctored with Photoshop).
In simple terms. The model gets trained to identify what a real improvement in wrinkles looks like, learning how to imitate both the before and after, and then uses this data to generate the resulting before and after images. The people in the images are not real — but the representation of the results being portrayed — the improvement in wrinkles — are real.
GANs can also be used to create videos and audio recordings. For example, a company could use a GAN to create a video ad that shows a person using the product and seeing results within minutes. The video would be generated by the GAN, based on real data samples. The resulting video would be more realistic and believable than a video created by traditional means.
Audio recordings can also be generated by GANs. For example, a company could use a GAN to create an audio recording of a person talking about how the product has helped them reduce wrinkles. The audio recording would be generated by the GAN, based on real data samples. The resulting audio would be more realistic and believable than an audio recording created by traditional means. The GAN could even be used to reproduce an audio clip with augmentations applied — like making the recording sound like it was recorded in a rain forest instead of a sound proofed studio booth.
GANs offer a powerful new tool for marketers to create realistic and believable ads. As more companies adopt GANs for marketing purposes, we are likely to see a surge in the number of new and innovative ads.
How could they be used to create more realistic and effective ads?
By training a GAN to generate realistic images of people or products, advertisers can create ads that are more likely to catch attention and be effective.
GANs can also be used to create more realistic ads by generating new images that are close to the target audience’s preferences. For example, if an advertiser knows that a certain consumer group likes images with blue skies and green trees, the advertiser can train a GAN to generate such images.
In addition, GANs can be used to create more realistic animations of people or products in ads. By generating a sequence of images that show a person or product in various positions, an animation can be created that is much more realistic than a traditional video or image.
There are endless possibilities for how GANs can be used to create more realistic and effective ads. As the technology continues to develop, we will likely see even more amazing and realistic ads generated by GANs.
What ethical considerations are there with using Generative Adversarial Networks in advertising?
When it comes to the ethical considerations of using Generative Adversarial Networks (GANs) in marketing, there are a few key points to keep in mind. First and foremost, it’s important to be aware of the potential for data manipulation and misuse when using GANs. As such, it’s critical that marketers take steps to ensure that data collected via GANs is used ethically and responsibly.
It’s also not always clear who owns the generated data. The company that created the GAN could claim ownership, but the people whose images were used to train the GAN could also make a claim.
In addition, it’s important to consider the potential impact of GAN-generated marketing content on consumers. While GANs can be used to create realistic and believable images, videos, and other content, there is always the potential for this content to mislead or misinform consumers. As such, marketers need to be thoughtful about the use of GANs and only use them in ways that will ultimately benefit consumers.
Finally, it’s worth considering the potential long-term impact of using GANs in marketing. As GANs become more sophisticated and widespread, there is a risk that they could be used to create increasingly realistic and convincing fake news stories or other content. This could have a profound impact on society, so it’s important to be thoughtful about the use of GANs now in order to avoid any negative consequences down the road.
What are the potential risks and benefits of using Generative Adversarial Networks in advertising?
Generative Adversarial Networks (GANs) are a new type of artificial intelligence (AI) that are being used more and more in marketing. There are potential risks and benefits to using GANs in marketing. A few examples have been compiled below.
Some examples of the potential risks of using GANs in marketing include:
1. They could be used to create fake customer reviews or testimonials.
2. They could be used to create fake social media posts or comments.
3. They could be used to create false positive results in marketing research studies (e.g., by generating fake survey responses).
4. They could be used to generate targeted ads that are too good to be true (e.g., by creating fake customer profiles).
5. They could be used to generate fake news stories about a company or product.
Some examples of the potential benefits of using GANs in marketing include:
1. They could be used to create realistic 3D images or videos of products (e.g., for product demonstrations).
2. They could be used to create realistic simulations of customer interactions (e.g. for customer service training).
4. They could be used to create targeted ads that are more personalized and relevant to individual customers.
5. They could be used to help automate the creation of marketing content — minimizing the overall time it takes to execute typically time-intensive tasks. (e.g. by auto-generating large volumes of text descriptions or images for products).
Wrapping up
It’s exciting to think about all the potential applications of GANs in marketing and advertising. The future is largely unwritten in this space — but some companies are early movers and have already started to adopt GANs into their workflows to create more effective marketing campaigns.
When considering whether or not to use GANs in marketing, it’s important to weigh the potential risks and benefits. GANs offer a lot of potential, but there are also some risks that need to be considered. It’s important to be aware of these risks and benefits before using GANs in marketing so that you can make an informed decision about whether or not they are right for your business.
Ultimately, it’s up to each individual company to decide whether or not the use of GANs in marketing is right for them. I’m curious to see how their adoption grows over the coming months and years, and to see how they’re used in future. Although there are some risks associated with using GANs, I’m hopeful that the technology will be used for good and that the future is positive.
Skeptical about the abilities of a GAN? Don’t be. This entire article was generated by one.
Leave a Reply